How can AI accelerate drug discovery?
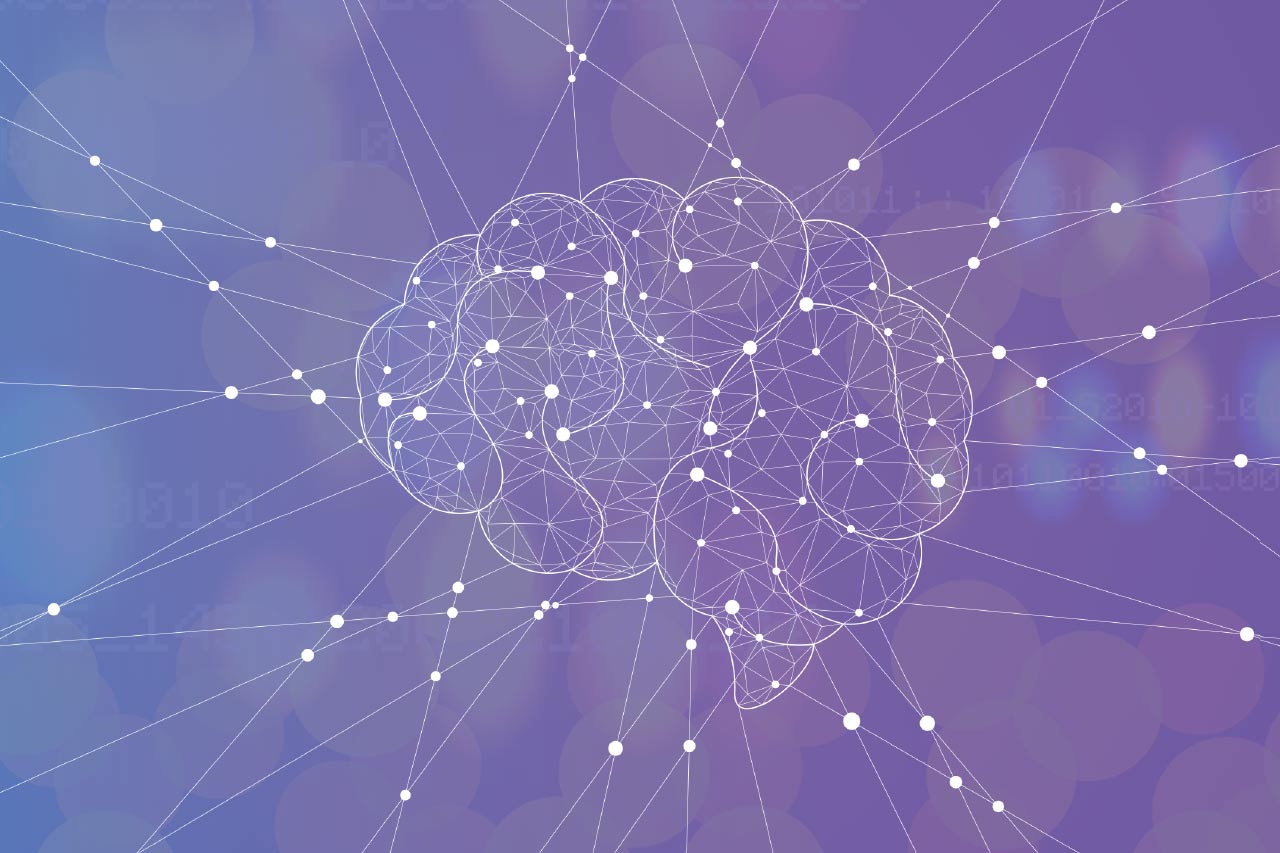
In February 2020, researchers from the Massachusetts Institute of Technology (MIT) announced the discovery of a new antibiotic, which showed to be efficient against several species of bacteria resistant to any other antibiotics, thanks to deep learning. In this context, at Alcimed, we wondered about the impact of the arrival of Artificial Intelligence (AI) on research and development (R&D) of new drugs. R&D is a long and expensive process. It is estimated that pharmaceutical companies spend an average of US$ 2.6 billion per drug developed and that it takes about 12 years to bring a new drug to market. Beyond cost and timing issues, a number of factors are pushing pharma companies to rethink their R&D model, such as the difficulty to come up with therapeutic solutions for rare diseases, the emergence of resistance to certain drugs, the development of personalized medicine, or pressure on the price of drugs. Thanks to the arrival of digital technologies in hospitals and research centers, data sources are multiplying, thus allowing AI to make its mark in the field of drug discovery.
Opportunities for the use of Artificial Intelligence in drug discovery
The use of databases for drug discovery is not new. However, the advent of AI could change the status quo because it is no longer essential for experts to understand pathophysiological mechanisms in order to imagine and identify new solutions. It is now sufficient to have enough data to train AI to identify the right correlations and find the new leads that the human eye could not see.
AI applications in the field of drug discovery include the selection of the most suitable therapeutic targets, the detection of active molecules on a given target, the identification of new indications for existing drugs, and the optimization of the medicinal chemistry of a drug candidate.
Discover the potential of artificial intelligence and the stakes of its implementation >
By building a model capable of recognizing chemical molecules with a lethal effect on E.coli, and then testing it on a database containing about 6,000 molecules whose effect on E.coli was not yet known, the MIT was able to select a new compound with antibiotic activity which had never been discovered before. Similarly, Iktos, a French startup working in the field of AI for drug discovery, applies generative models to public databases in order to design new molecules for a given target or specification.
AI to meet the challenges of accelerating research and reducing costs
The time spent searching for molecules of interest or therapeutic targets can be significantly reduced with the arrival of AI, which is one of its major advantages. In 2019, the identification of molecules to inhibit transmembrane receptors involved in fibrosis was achieved in only 21 days using a Generative Tensor Enhanced Learning Tool (GENTRL) developed by a biotechnology company, Insilico Medicine. This tool can identify and learn the properties of a chemical space, then virtually generate new molecules from that space, and identify those which best fit given specifications and target.
This time saving is a real advantage for pharmaceutical companies, which have already created partnerships with start-ups offering AI tools for drug discovery. With AI algorithms, the search for molecules of therapeutic interest could be performed 100 times faster than with more conventional tools, as estimated by Atomwise, a company specializing in the use of AI for drug discovery.
The costs associated with the research can also be reduced thanks to better targeting and prioritizing of the molecules of interest to be tested.
Current limitations in the use of AI in drug discovery
Machine learning models need a lot of data, and although many datasets on certain diseases or drugs are public, this is not always enough to feed AI algorithms. Key issues for the future will include ensuring more open access to more data, generation of missing data and optimization of existing AI solutions to require less data to function.
Legislative issues also arise in this context, such as access to patient data and the use of AI algorithms for drug discovery.
To sum up, AI has many potential applications for drug discovery, in particular by making it possible to discover yet unknown therapeutic targets and to design efficient molecules for a given target faster and with a better probability of success in clinical trials than with more conventional research methods. Even though AI tools are increasingly numerous and diverse, there is no magic solution relevant to any type of project. The challenge for pharmaceutical companies is therefore to understand which technologies are best suited to a given problem and what are the conditions for using them.
About the authors
Axelle, Consultant in the Alcimed Healthcare team in France
Amélie, Head of the Data activity in Alcimed’s Healthcare team in France