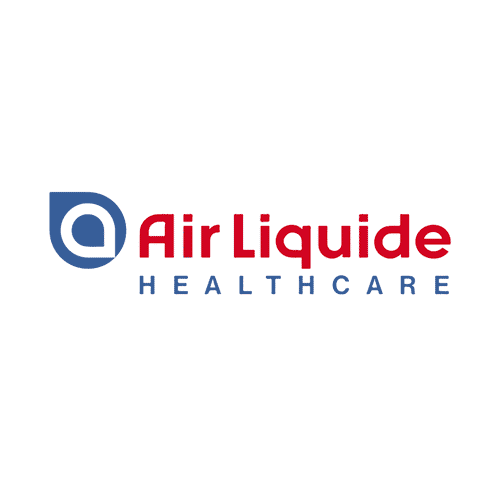
Predictive modeling
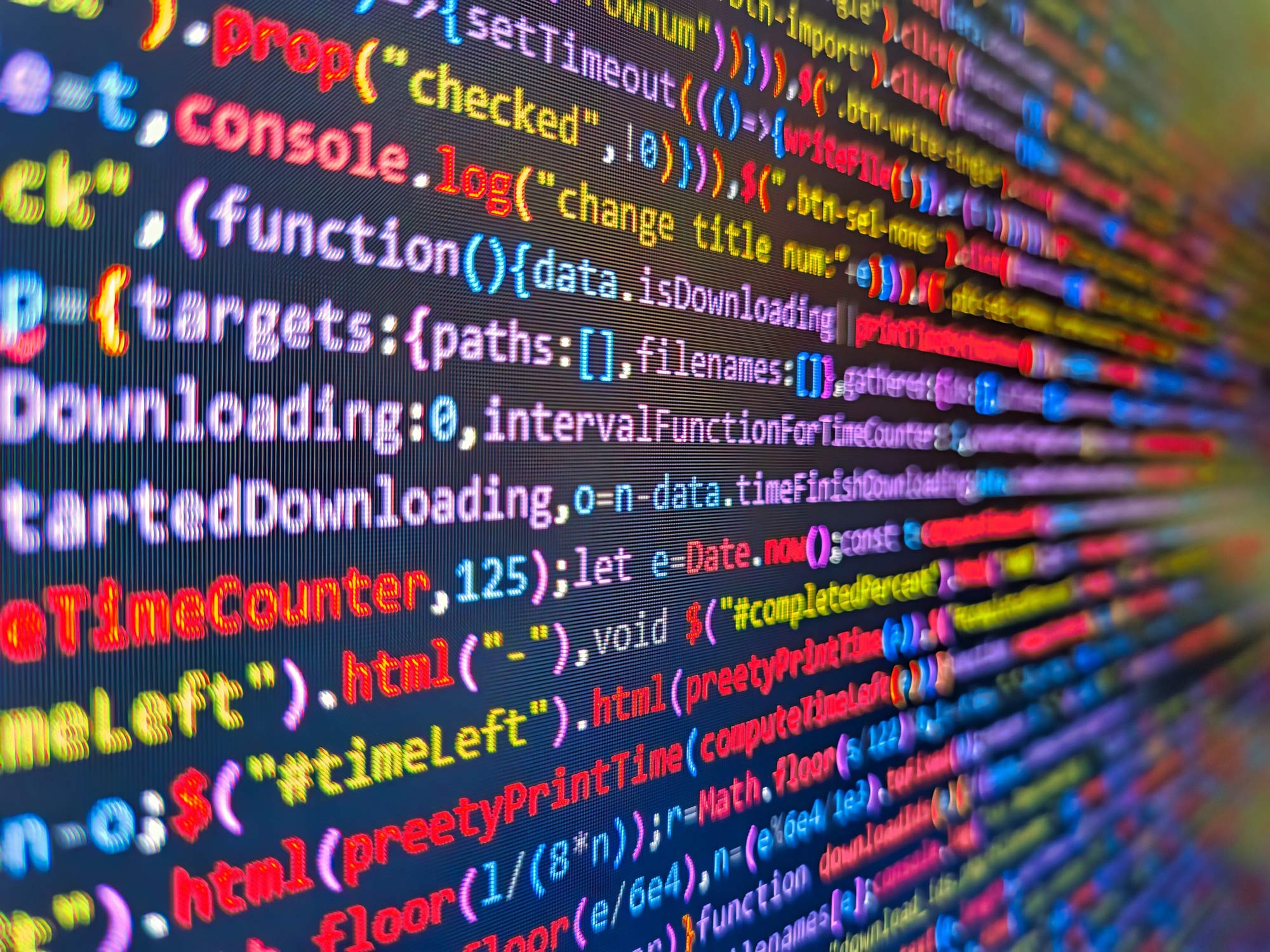
Anticipate trends and behaviors with machine learning
Our specialized team supports companies in building their predictive models through the development of data mining algorithms or predictive analysis of internal and external data using models ranging from linear regression to neural networks.
They trust us
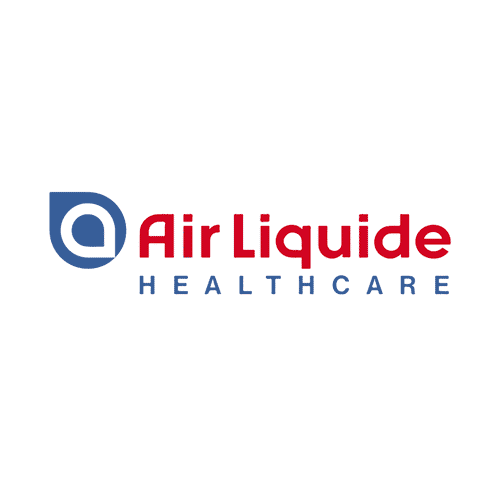
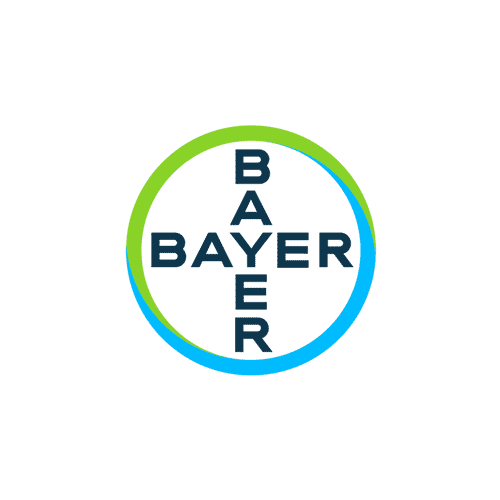
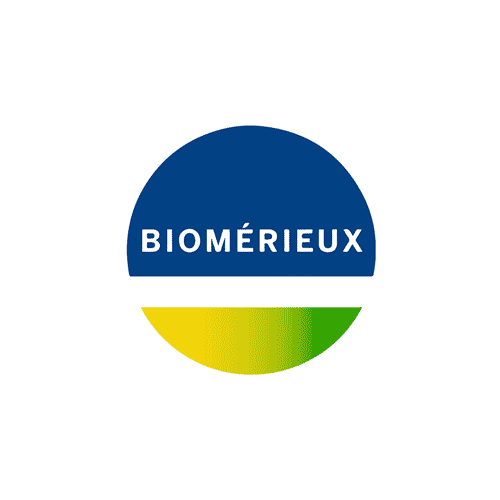
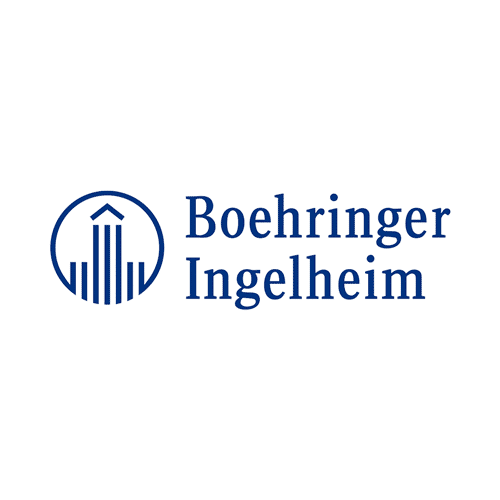
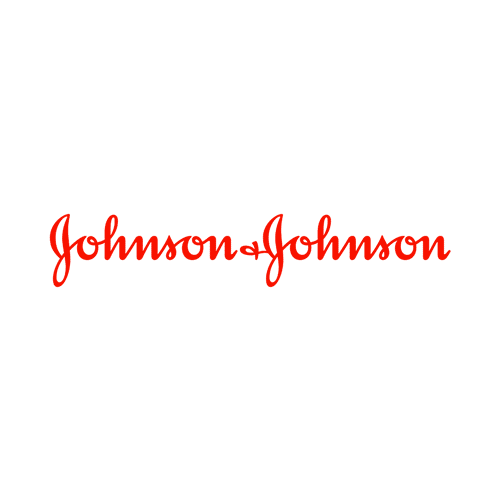
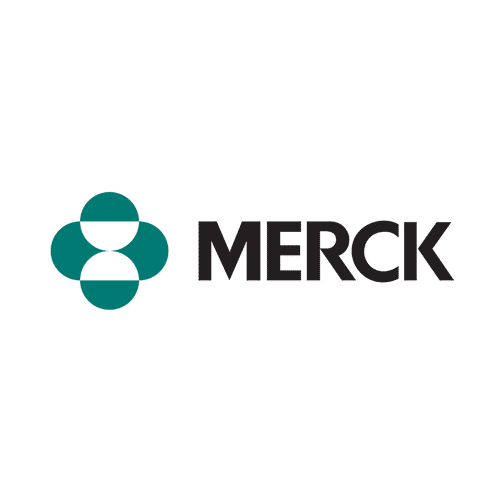
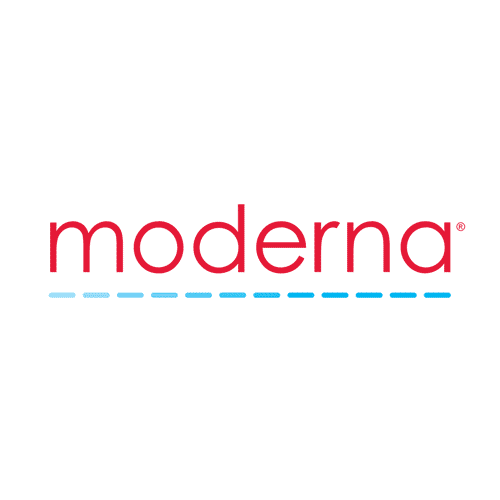
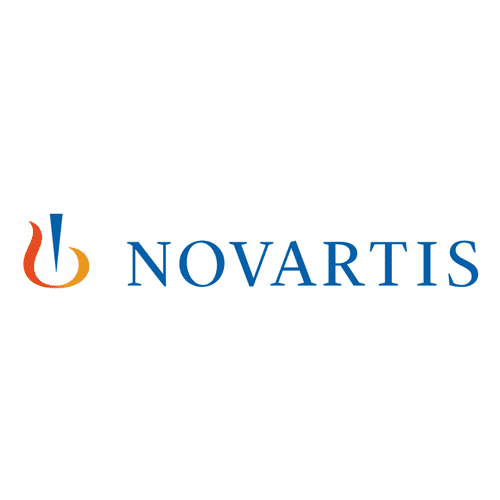
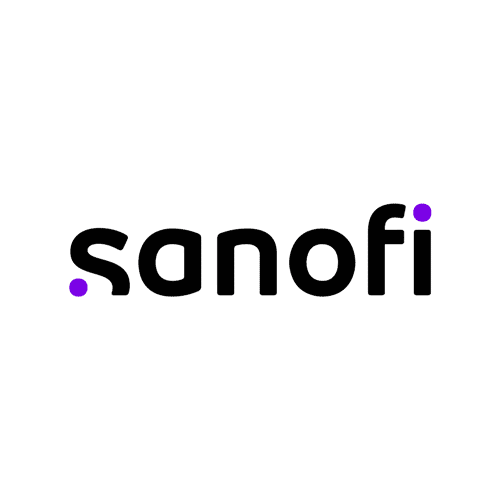
The challenges related to predictive modeling
Predictive models are tools to help make decisions regarding trends and future behaviors, to improve operational efficacy, to reduce costs, to minimize all types of risk, and more generally to stay competitive on a market.
Numerous challenges must be considered to ensure the right quality of a predictive analysis. Amongst these are choosing an adequate algorithm, defining the right parameters and calibrations, and collecting sufficiently representative training data in high quantity.
Before diving into the construction of a predictive model, it is imperative to rethink your business use case to better define the goal and the manner in which the model should be used by your operational teams. Indeed, depending on the nature of your goal (qualitative or quantitative), it will be necessary to select between classification or regression algorithms.
For example, to optimize your business’s sales, regression models will allow you to predict the effects of new marketing campaigns on your part of the market (based on historical observations), while classification models could help you better segment your customer base and better guide your commercial strategy. Once the choice has been made, it’s necessary to know which theoretical model is best adapted to your specific problem.
There are a multitude of algorithms in each of these two categories, and making the right choice is not always simple: choosing between a linear regression or polynomial or logistic regression, choosing between a decision tree or an SVM or a neural network, and other similar questions can complicate the decision. The technical characteristics of these techniques makes them more or less suitable, not only for your subject (types of input data, number of dimensions, expected outcomes, etc.), but also for your specific needs (for example, rapidity and power of prediction).
The capacity to interpret the results from the algorithm is an essential aspect to integrate into the specifications of most solutions in business case usages, as our object isn’t to introduce technical opacity into your processes, but rather to make them simpler and ensure that the model is used in the daily operations of your company.
Identifying the right algorithm thus requires both technical expertise and a solid knowledge and understanding of the businesses challenges.
How can we select the most suitable model for our problems and analytical needs?
Once the right algorithm has been selected, another technical challenge is adapting the parameters and calibrations to avoid over-adaptation of the model to the existing data, which is referred to as “overfitting”. The quality of the model is tested by different indicators that keep track of the reliability of the prediction, such as the precision (rate of correct detection), the sensitivity (ability to correctly detect “truth”), and the specificity (ability to detect “false”).
Trying to maximize these indicators can lead to the inclusion of an enormous amount of variables in predictive analysis, or to use increasingly complex models. It is important to keep a portion of the dataset to test the model on, and not to train it on. Since training data are often more homogenous than real-life data, it is also important to limit the complexity of the machine learning model to the minimum required. Compiling the results from multiple different models is a technique that can limit the inherent biases of each of these models.
How can one ensure the quality of their parameters? How can a forecasting model be adapted in order to anticipate scenarios linked to events that have never happened?
During the model’s calibration and training, the inputted data are also a critical issue. Beyond the quantity of data (which is one of the principal challenges when building a machine learning model), their quality and representativeness are also key to be able to draw relevant conclusions. Specifically, unbalanced data can bias the model’s training. If one wishes to train an algorithm to classify images of cats and dogs based on 1000 pictures of cats and 100 of dogs, the notion of a greater frequency of occurrence of cats will come out in the classification of new images.
This imbalance can be easy to identify if it concerns the principal object of detection, but is more difficult if it is based on one element amongst others, for example an over-representation of kittens amongst the images. Historic databases can be biased, such as clinical trial databases in which white men are over-represented with respect to the general population. During data collection, it’s important to fix and correct for these biases in our data sources by reducing the size of the over-represented sample (undersampling) or artificially increasing the size of the under-represented sample (oversampling).
How can we improve our selection of inputted data sources to avoid biasing the training of the algorithms?
Beyond the upstream cleaning of the inputted data sources, it is often necessary to later clean the mass-collected data. This critical step in any data science process determines the success of the prediction on the analytical level, and also its value on the interpretive level. Doing so can make it necessary to make decisions in order to improve the signal-to-noise ratio, which can result in eliminating part of the signal. Specifically, the domain of data science focused on the analysis of textual data, Natural Language Processing (NLP), can require particularly robust data cleaning depending on the source used. The collection of information from social media, for example, requires much work in order to succeed in detecting and interpreting mis-spelled words or abbreviations.
Which data are sufficiently rich for their analysis to bring value? How can we draw value from our internal or external databases?
How we support you in your projects related to predictive modeling
For nearly 30 years, Alcimed has supported industry leader, institutional, SME, and innovative start-up in their projects of innovation and developing new markets.
Skilled in the field and competencies of data science thanks to our dedicated team, we offer personalized support to the senior management and business unit managers (marketing, commercial affaires, operational excellence, etc.) in numerous activity sectors (healthcare, agri-food, energy and mobility, chemistry and materials, cosmetics, aerospace and defense, etc.), where we help you identify the business-specific challenges for which analytical predictions can provide a reliable and solid answer.
Our data science team supports you in each step of your project, from identifying use cases to implementing a predictive model and reflecting on its implications. This includes selection of the right model, the parameters, mining and cleaning of both internal and external data, and the presentation of results in an ergonomic manner. You can count on our expertise to bring your project to a successful conclusion with concrete outcomes!
Examples of recent projects carried out for our clients in predictive modeling
Analytical prediction of the number of construction permits in the pipeline
To support our client, a leading construction and public works player, in predicting its business volume, Alcimed developed a machine learning algorithm to predict, based on historical public data and before they are all officially referenced by the local authorities, the total number of building permits filed in the current month.
This project enabled the client to anticipate its sales forecast and to adapt several of their activities in advance.
Predictive model for detecting weak signals in a body of messages
Alcimed supported the French sector of an international pharmaceutical player in the definition, design, and implementation of data visualization tools for the data collected by its Medical Information Database, allowing the team to monitor the unusual and future concerns of healthcare professionals.
Our team implemented LNP techniques and an advanced statistical analysis of queries, allowing the automatic detection of infrequently mentioned themes and words that had potential to become major future subjects.
We also supported the deployment of this approach in the product team and in our client’s systems.
Predictive analysis of the potential launch of an oncology product
Alcimed supported a major healthcare industry player in modeling a business case to evaluate the opportunity for launching a new oncology product in 6 key markets over the next 15 years.
Our team collected epidemiology information and data on the usage rates of different health products that are available or under development to create a model of the evolution of the market size and the market shares in the concerned geographies.
We could thereby predict the future performances of a new health product launch thanks to time series analysis techniques.
Development of a predictive model for risk classification for a financial services provider
Our client, a financial services provider, wanted to investigate the potential of AI models for the prediction of risk on their investment projects.
Our work started by a mapping of the data available for predictions, followed by an extract, transform, and load (ETL) process for model building. Our team then assessed multiple models in an iterative process until a final model was selected based on its performance.
Finally, our team adapted the model so it can be put in production and adapted to the client’s infrastructure who could then use it for a better decision making on new projects.
Development of a predictive model for the energy efficiency of lightbulbs
For an energy player, Alcimed was asked for support to leverage data from performance evaluations of various energy-efficient light bulbs. These evaluations included a secondary measure: the energy efficiency of the bulbs tested. The goal was to utilize these performance tests to predict the energy efficiency of future light bulb designs.
To do so, our data team extracted and compiled the energy consumption data and design specifications of each light bulb studied in the performance evaluations. After preprocessing and standardization of data, we developed a predictive model using this processed data to estimate the energy efficiency of a given light bulb design.
Thanks to this model, it is now possible for our client to predict the energy efficiency of a light bulb by knowing its design specifications. This tool enables to save time and resources in new product development and our client can now take full advantage of data from previous performance evaluations.
You have a project?
To go further
Data - AI
AI in medical imaging, a revolution in medical diagnosis and patient care
Artificial intelligence has made its mark in many specialties, such as medical imaging. But how is AI in medical imaging used, and what are the challenges ahead?
Healthcare
Personalized medicine: molecular testing to enhance the prostate cancer patient pathway
Personalized medicine: Recent advancements in molecular testing capabilities are beginning to impact the treatments landscape of prostate cancer by making it more targeted.
Data - AI
Computer Vision in Healthcare : the applications and challenges of this new AI solution
Computer Vision is making its way into the healthcare sector, with a variety of medical AI solutions. But how is it being used, and what are the challenges ahead?
Founded in 1993, Alcimed is an innovation and new business consulting firm, specializing in innovation driven sectors: life sciences (healthcare, biotech, agrifood), energy, environment, mobility, chemicals, materials, cosmetics, aeronautics, space and defence.
Our purpose? Helping both private and public decision-makers explore and develop their uncharted territories: new technologies, new offers, new geographies, possible futures, and new ways to innovate.
Located across eight offices around the world (France, Europe, Singapore and the United States), our team is made up of 220 highly-qualified, multicultural and passionate explorers, with a blended science/technology and business culture.
Our dream? To build a team of 1,000 explorers, to design tomorrow’s world hand in hand with our clients.
Predictive models are built on the analysis of past and present data, the goal of which is to predict future events or outcomes. As such, predictive models theorize the future evolution of a variable by identifying the patterns in a large collection of historical data (often referred to as Big Data) obtained by data mining from diverse sources.
This identification is now done in an automatic manner with algorithms and theoretical statistical models, such as linear regression, decision trees, k-means clustering, neuronal networks, or other machine learning techniques.
On the one hand, predictive modeling involves the creation of statistical models that exploit a database to predict an outcome. Adjustments and improvements must be made manually.
On the other hand, machine learning is an approach to artificial intelligence in which algorithms learn patterns or actions from data without being explicitly programmed. A process of learning and improvement takes place automatically.Predictive modeling can be done using machine learning methods, but machine learning involves a broader set of techniques with more varied purposes (prediction, clustering, regression, anomaly detection, etc.).